Mass balance parameter perturbation experiments with OGGM#
By now, we assume that you have read and run the mass balance calibration tutorial. We know that it is a lot of new information to take in!
In this notebook, we will:
re-iterate and discuss the important role that mass balance calibration plays in the projections
give you some tools to run parameter perturbation experiments (this will also illustrate useful aspects of the OGGM internals)
provide some keys about how to address the calibration process in your use case
Set-up#
import matplotlib.pyplot as plt
import matplotlib
import pandas as pd
import xarray as xr
import numpy as np
import os
import oggm
from oggm import cfg, utils, workflow, tasks, graphics
from oggm.core import massbalance
from oggm.core.massbalance import mb_calibration_from_scalar_mb, mb_calibration_from_geodetic_mb, mb_calibration_from_wgms_mb
cfg.initialize(logging_level='WARNING')
cfg.PATHS['working_dir'] = utils.gettempdir(dirname='OGGM-calib-pertubation', reset=True)
cfg.PARAMS['border'] = 80
2024-04-25 13:37:36: oggm.cfg: Reading default parameters from the OGGM `params.cfg` configuration file.
2024-04-25 13:37:36: oggm.cfg: Multiprocessing switched OFF according to the parameter file.
2024-04-25 13:37:36: oggm.cfg: Multiprocessing: using all available processors (N=4)
We start from our two well known glaciers in the Austrian Alps, Kesselwandferner and Hintereisferner. But you can also choose any other other glacier, e.g. from this list.
# we start from preprocessing level 5
base_url = 'https://cluster.klima.uni-bremen.de/~oggm/gdirs/oggm_v1.6/L3-L5_files/2023.3/elev_bands/W5E5/'
gdirs = workflow.init_glacier_directories(['RGI60-11.00787', 'RGI60-11.00897'], from_prepro_level=5, prepro_base_url=base_url)
2024-04-25 13:37:38: oggm.workflow: init_glacier_directories from prepro level 5 on 2 glaciers.
2024-04-25 13:37:38: oggm.workflow: Execute entity tasks [gdir_from_prepro] on 2 glaciers
Changing the mass balance parameters for the mass balance model#
We just downloaded the data. Let’s have a look at the calibrated parameters for these glaciers:
# Pick each glacier
gdir_kwf = gdirs[0]
gdir_hef = gdirs[1]
gdir_hef.read_json('mb_calib')
{'rgi_id': 'RGI60-11.00897',
'bias': 0,
'melt_f': 5.0,
'prcp_fac': 3.5331695984128135,
'temp_bias': 1.7583130779353044,
'reference_mb': -1100.3,
'reference_mb_err': 171.8,
'reference_period': '2000-01-01_2020-01-01',
'mb_global_params': {'temp_default_gradient': -0.0065,
'temp_all_solid': 0.0,
'temp_all_liq': 2.0,
'temp_melt': -1.0},
'baseline_climate_source': 'GSWP3_W5E5'}
gdir_kwf.read_json('mb_calib')
{'rgi_id': 'RGI60-11.00787',
'bias': 0,
'melt_f': 5.0,
'prcp_fac': 2.96122858393561,
'temp_bias': 1.7583130779353044,
'reference_mb': -514.8000000000001,
'reference_mb_err': 136.3,
'reference_period': '2000-01-01_2020-01-01',
'mb_global_params': {'temp_default_gradient': -0.0065,
'temp_all_solid': 0.0,
'temp_all_liq': 2.0,
'temp_melt': -1.0},
'baseline_climate_source': 'GSWP3_W5E5'}
These parameters are stored in a file called mb_calib.json
in the glacier directory. This file is then read by the mass balance model when created:
mbmod = massbalance.MonthlyTIModel(gdir_hef)
mbmod.calib_params
{'rgi_id': 'RGI60-11.00897',
'bias': 0,
'melt_f': 5.0,
'prcp_fac': 3.5331695984128135,
'temp_bias': 1.7583130779353044,
'reference_mb': -1100.3,
'reference_mb_err': 171.8,
'reference_period': '2000-01-01_2020-01-01',
'mb_global_params': {'temp_default_gradient': -0.0065,
'temp_all_solid': 0.0,
'temp_all_liq': 2.0,
'temp_melt': -1.0},
'baseline_climate_source': 'GSWP3_W5E5'}
Therefore, if you want to mess around with these parameters, “all you have to do” is to overwrite this file somehow, or create a new one and ask the mass balance model to read it instead of the default one. Let’s do that:
params = gdir_hef.read_json('mb_calib')
params['melt_f'] = 7 # a new value
gdir_hef.write_json(params, 'mb_calib', filesuffix='_perturbed') # write a new file, with perturbed parameters
We can read it in with:
mbmod_perturbed = massbalance.MonthlyTIModel(gdir_hef, mb_params_filesuffix='_perturbed')
mbmod_perturbed.calib_params
{'rgi_id': 'RGI60-11.00897',
'bias': 0,
'melt_f': 7,
'prcp_fac': 3.5331695984128135,
'temp_bias': 1.7583130779353044,
'reference_mb': -1100.3,
'reference_mb_err': 171.8,
'reference_period': '2000-01-01_2020-01-01',
'mb_global_params': {'temp_default_gradient': -0.0065,
'temp_all_solid': 0.0,
'temp_all_liq': 2.0,
'temp_melt': -1.0},
'baseline_climate_source': 'GSWP3_W5E5'}
Just for fun, check what this means for the mass balance:
h = np.linspace(2000, 3800, 80)
mb_default = mbmod.get_annual_mb(h, year=1980) * cfg.SEC_IN_YEAR * cfg.PARAMS['ice_density']
mb_perturbed = mbmod_perturbed.get_annual_mb(h, year=1980) * cfg.SEC_IN_YEAR * cfg.PARAMS['ice_density']
plt.plot(mb_default, h);
plt.plot(mb_perturbed, h);
plt.xlabel('Mass balance (mm w.e)'); plt.ylabel('Elevation');
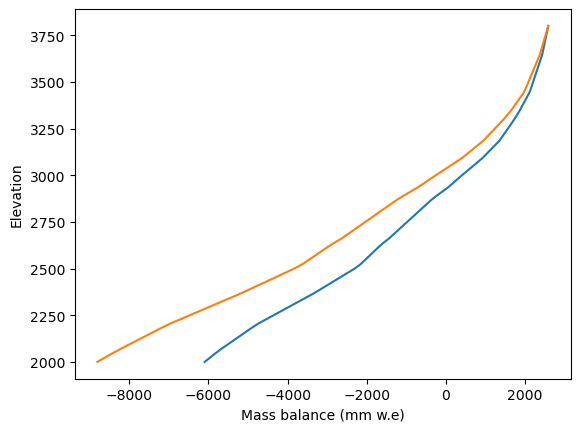
So far so good. But how to feed this into the heavy OGGM pipeline? Many OGGM users will be more familiar with the run_*
entity tasks. These tasks “hide” the process of creating the mass balance model and therefore make it look like we cant’s change anything internally.
We could have added a mechanism to pass the mb_params_filesuffix
from, for example, run_random_climate
, to the underlying mass balance model (similar to the “climate_input_filesuffix” mechanism). We may add this one day, but for now I’d like to use this opportunity to demonstrate another possible mechanism:
# So far so good: default run with the default mass balance
tasks.run_random_climate(gdir_hef, y0=2000, nyears=100, seed=1, output_filesuffix='_default');
# Let' create another "mass balance model" which is like the default one but with another default parameter
from functools import partial
PerturbedMassBalance = partial(massbalance.MonthlyTIModel, mb_params_filesuffix='_perturbed')
# Pass it to the run task
tasks.run_random_climate(gdir_hef, y0=2000, nyears=100, seed=1, mb_model_class=PerturbedMassBalance, output_filesuffix='_perturbed');
The partial function allows to create a function that is created by fixing a certain number of arguments of another function. Here we create a new “class” which is the same as the default original one, but by setting one parameters to another value. This proves very useful here, since we are just tricking OGGM into using the new one!
Let’s check the outcome:
with xr.open_dataset(gdir_hef.get_filepath('model_diagnostics', filesuffix='_default')) as ds:
ds_default = ds.load()
with xr.open_dataset(gdir_hef.get_filepath('model_diagnostics', filesuffix='_perturbed')) as ds:
ds_perturbed = ds.load()
ds_default.volume_m3.plot(label='Default');
ds_perturbed.volume_m3.plot(label='Perturbed');
plt.legend();
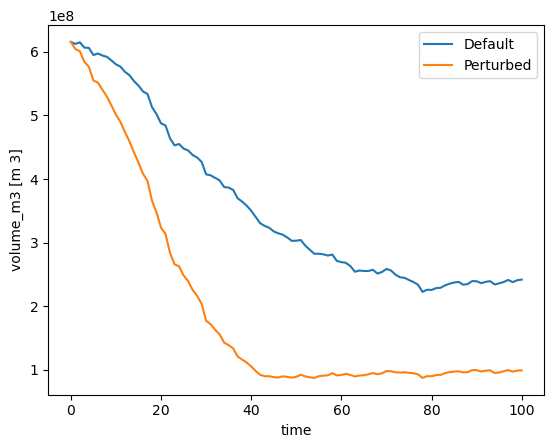
Quite a big difference for “just” 2 units of melt factor more, from 5 to 7!
More structured parameters perturbation experiments#
OK, so let’s say we want to do this “at scale”. We actually had such an assignment recently for the PROTECT SLR project. We were asked to do a number of perturbed simulations with parameters diverging from their default values, for example +1 temp_bias everywhere. But how to do this, knowing that each glacier has a different temp_bias? We can’t simply set the bias to 1 everywhere (we need +=1).
For this I wrote a “task”, originally outside of OGGM but that is now (v1.6.4) part of the main codebase. Let’s have a look at it:
@entity_task(log, writes=['mb_calib'])
def perturbate_mb_params(gdir, perturbation=None, reset_default=False, filesuffix=''):
"""Replaces pre-calibrated MB params with perturbed ones for this glacier.
It simply replaces the existing `mb_calib.json` file with an
updated one with perturbed parameters. The original ones
are stored in the file for re-use after perturbation.
Users can change the following 4 parameters:
- 'melt_f': unit [kg m-2 day-1 K-1], the melt factor
- 'prcp_fac': unit [-], the precipitation factor
- 'temp_bias': unit [K], the temperature correction applied to the timeseries
- 'bias': unit [mm we yr-1], *substracted* from the computed MB. Rarely used.
All parameter perturbations are additive, i.e. the value
provided by the user is added to the *precalibrated* value.
For example, `temp_bias=1` means that the temp_bias used by the
model will be the precalibrated one, plus 1 Kelvin.
The only exception is prpc_fac, which is multiplicative.
For example prcp_fac=1 will leave the precalibrated prcp_fac unchanged,
while 2 will double it.
Parameters
----------
perturbation : dict
the parameters to change and the associated value (see doc above)
reset_default : bool
reset the parameters to their original value. This might be
unnecessary if using the filesuffix mechanism.
filesuffix : str
write the modified parameters in a separate mb_calib.json file
with the filesuffix appended. This can then be read by the
MassBalanceModel for example instead of the default one.
Note that it's always the default, precalibrated params
file which is read to start with.
"""
df = gdir.read_json('mb_calib')
# Save original params if not there
if 'bias_orig' not in df:
for k in ['bias', 'melt_f', 'prcp_fac', 'temp_bias']:
df[k + '_orig'] = df[k]
if reset_default:
for k in ['bias', 'melt_f', 'prcp_fac', 'temp_bias']:
df[k] = df[k + '_orig']
gdir.write_json(df, 'mb_calib', filesuffix=filesuffix)
return df
for k, v in perturbation.items():
if k == 'prcp_fac':
df[k] = df[k + '_orig'] * v
elif k in ['bias', 'melt_f', 'temp_bias']:
df[k] = df[k + '_orig'] + v
else:
raise InvalidParamsError(f'Perturbation not valid: {k}')
gdir.write_json(df, 'mb_calib', filesuffix=filesuffix)
return df
It’s a fairly easy piece of code isn’t it? Let’s apply it in a latin hypercube parameter set where we change all parameters in a structured way:
from scipy.stats import qmc
sampler = qmc.LatinHypercube(d=3)
sample = sampler.random(n=30)
def log_scale_value(value, low, high):
"""This is to sample multiplicative factors in log space to avoid assymetry (detail, but important)."""
return 2**((np.log2(high) - np.log2(low))*value + np.log2(low))
sample[:,0] = 4*sample[:,0] - 2 # DDF factor (melt_f): apply change [-2, +2] mm/(°C day)
sample[:,1] = 2*sample[:,1] - 1 # temperature bias (temp_bias): apply change [-1, +1] °C
sample[:,2] = log_scale_value(sample[:,2], 0.5, 2) # precipitation scaling factor (prcp_fac): apply scaling [0.5, 2] on log2
params_df = pd.DataFrame(sample, columns=['melt_f', 'temp_bias', 'prcp_fac'])
params_df.plot.scatter(x='temp_bias', y='prcp_fac');
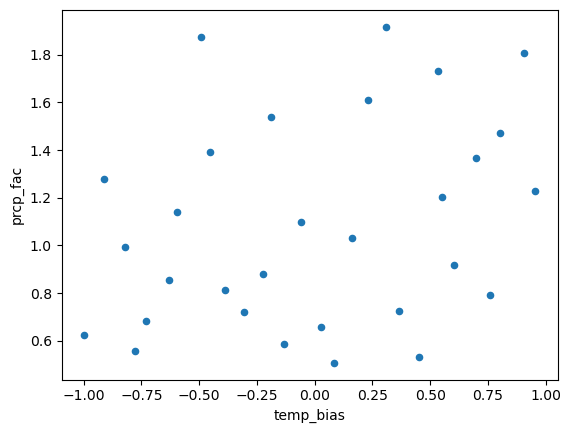
cfg.set_logging_config('CRITICAL') # shut down log output (bad!)
cfg.PARAMS['continue_on_error'] = True
for exp in range(len(params_df)):
params = params_df.loc[exp]
# clim_params = {k: params[k] for k in ('temp_bias', 'prcp_fac', 'melt_f')}
exp = f'{exp:02d}'
workflow.execute_entity_task(tasks.perturbate_mb_params, gdirs, perturbation=params, filesuffix=f'_{exp}')
PerturbedMassBalance = partial(massbalance.MonthlyTIModel, mb_params_filesuffix=f'_{exp}')
workflow.execute_entity_task(tasks.run_random_climate, gdirs,
y0=2000,
nyears=100,
seed=1,
mb_model_class=PerturbedMassBalance,
output_filesuffix=f'_{exp}', # recognize the run for later
);
out_df = pd.DataFrame()
for exp in range(len(params_df)):
try:
ds = utils.compile_run_output(gdirs, input_filesuffix=f'_{exp:02d}')
if np.any(ds.volume.isnull()):
continue
out_df[f'{exp:02d}'] = ds.volume.sum(dim='rgi_id').to_series()
except RuntimeError:
pass
out_df.plot(legend=False, color='k', alpha=0.5);
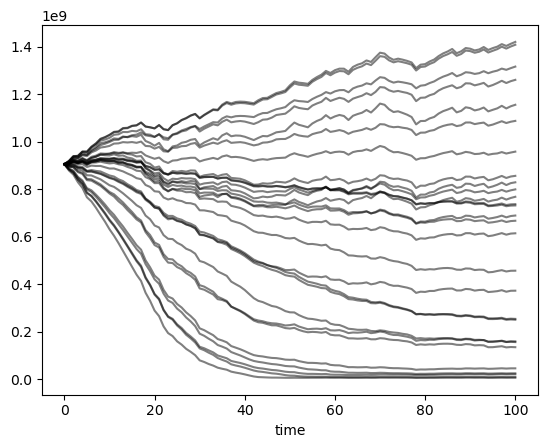
Parameters perturbation experiments which match observations#
The section above is nice, but works only for a problem setting where we don’t ask the mass balance model to match observations. If we were to match obervations, things would be quite different!
To do this, we could define a new task very much like the above, but this time realizing a calibration step before writing its solution down.
This exercise is left to the reader ;-)
What’s next?#
Check out the massbalance_global_params.ipynb notebook
return to the OGGM documentation
back to the table of contents